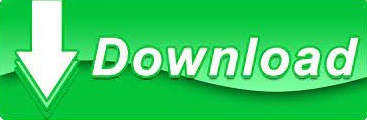

We’ll have more to say about this in a moment.Īnother way of looking at ANCOVA is to remove the covariate from the analysis (see Figure 4).įigure 4 – Reduced ANCOVA model for Example 1 Where is the mean for training method adjusted to remove the effect of the covariate. This is equivalent to rejecting the following null hypothesis: We also see that differences in training are significant (p-value =. 05), and so family income is significant in predicting reading scores.
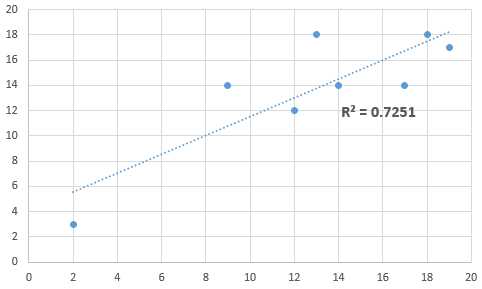
The sum squares are calculated as follows (the degrees of freedom are similar):įrom Figure 3, we see that the covariate is significant (p-value = 0.000006 <.
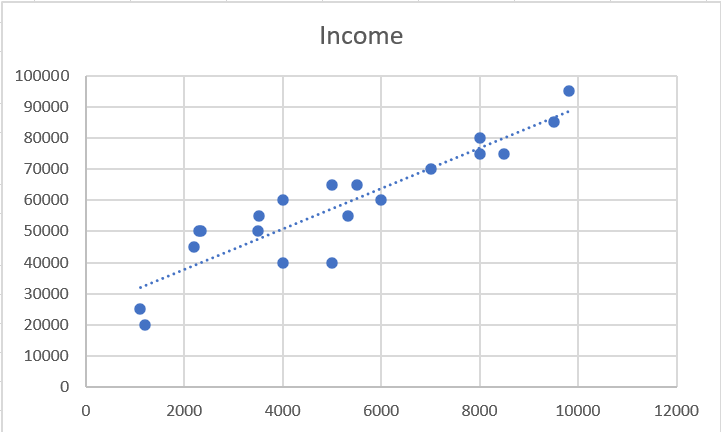
The first model, shown in Figure 3, is essentially the full model with the variation due to the covariate identified. The ANCOVA model follows directly from Figure 2. Running Excel’s regression data analysis tool for each model we obtain the results displayed in Figure 2 (excluding the complete model, which we will look at later):įigure 2 – Full and reduced regression models Combining Models Partial model ( x, t) – only income and treatments are used.Partial model (y, x) – only reading scores and income are used.Partial model (y, t ) – only reading scores and treatments are used.Full model (y, x, t) – all the variables are used, interaction of treatment with income is not modeled.Complete model (y, x, t, x*t) – all the variables are used, interaction between treatments and income is modeled.Now we define the following regression models: Thus the data from Figure 1 of Basic Concepts of ANCOVA takes the form for regression analysis shown in Figure 1.įigure 1 – Data for Example 1 along with dummy variables Different Regression Models As we have done several times (see ANOVA using Regression), we use dummy variables for the treatments (i.e. Our objective is to analyze the effect of teaching method, but without the confounding effect of family income (the covariate). Example 1: Carry out the analysis for Example 1 of Basic Concepts of ANCOVA using a regression analysis approach.
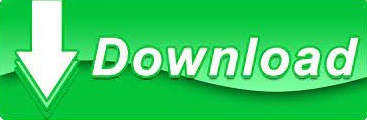